Are you looking for information regarding the Google Cloud Certified Professional Data Engineer exam? If yes, then you are at the right place. We at Testprep training are going to explore the Google Cloud Certified Professional Data Engineer exam in this article. Let us just start with the Professional Data Engineer. What exactly the Professional Data Engineer is? A Professional Data Engineer supports decision-making through data collection, transformation, and distribution. They need to design, develop, operate, secure, and monitor data processing systems with a focus on security, scalability, reliability, and flexibility. Additionally, they should be able to use, implement, and continually refine existing machine learning models.
The Professional Data Engineer exam assesses your ability to:
- Design data processing systems
- Ingest and process the data
- Store the data
- Prepare and use data for analysis
- Maintain and automate data workloads
The Google Cloud Certified Professional Data Engineer is a widely known and tough IT certification test. This exam, at an expert level, can elevate your chances of landing a prestigious job in a reputable organization. While it’s a highly esteemed certification, it’s also quite challenging due to the extensive and in-depth knowledge that Google requires you to have.
GCP Data Engineer Exam Details
First of all, let us discuss the details of the Google Cloud Certified Professional Data Engineer certification exam. In the Google Cloud Certified Professional Data Engineer exam, candidates are given a time limit of 2 hours to complete the test. Additionally, the exam questions are in the format of multiple-choice and multiple-select. To pass the exam, the candidate needs to achieve a score of 70%. The certification is valid for two years and can be taken in four languages: English, Japanese, Spanish, and Portuguese. The exam fee is $200 USD. Since each exam has its own set of requirements, it’s important to be aware of the specific requirements for the Professional Data Engineer exam, such as:
- The candidate should possess scalability and efficiency.
- S/he should be able to design and monitor data processing systems with a particular emphasis on security.
- Above all, a data engineer should be able to leverage and continuously train pre-existing machine learning models.
Scheduling the exam
Once the candidate comprehends the essence of the Professional Data Engineer certification, the next step is to enroll for the exam. If the candidate is determined to pursue this certification and become a certified Google Data Engineer, they should proceed to register for the exam and commence the preparation. Here are the steps to apply for the exam:
To book the exam, the candidate can go to the Official Google Cloud website.
- The candidate will need a Web assessor account. They are supposed to create one in order to register themselves for the exam. To create, click here
- Create the account with their personal email address and not their work address.
- Check the catalog and register for the exam they want to apply for.
- Choose the exam center i.e. Kryterion Testing Centre.
- Upon registering for the exam, candidates must schedule an exam time at a Kryterion testing center that suits their convenience. They can locate the nearest testing center here.
Furthermore, if the candidate doesn’t pass the certification exam, they can take it again after 14 days. Similarly, if you don’t pass the second time, you must wait 60 days. Further, if they don’t pass the third attempt, they will have to wait a year before trying again. Above all, payment is required each time they take an exam. It’s crucial to remember that all Google Cloud certifications remain valid for two years from the certification date. To uphold their certification status and certificate number, candidates need to recertify their certificates within this period.
Course Structure
Now that we have a clearer understanding of the necessary information, let’s delve into the exam outline. We’ll take a look at the topics essential for the exam, focusing on the Google Cloud Certified Professional Data Engineer Syllabus.
Section 1: Designing data processing systems (22%)
1.1 Designing for security and compliance. Considerations include:
- Identity and Access Management (e.g., Cloud IAM and organization policies) (Google Documentation: Identity and Access Management)
- Data security (encryption and key management) (Google Documentation: Default encryption at rest)
- Privacy (e.g., personally identifiable information, and Cloud Data Loss Prevention API) (Google Documentation: Sensitive Data Protection, Cloud Data Loss Prevention)
- Regional considerations (data sovereignty) for data access and storage (Google Documentation: Implement data residency and sovereignty requirements)
- Legal and regulatory compliance
1.2 Designing for reliability and fidelity. Considerations include:
- Preparing and cleaning data (e.g., Dataprep, Dataflow, and Cloud Data Fusion) (Google Documentation: Cloud Data Fusion overview)
- Monitoring and orchestration of data pipelines (Google Documentation: Orchestrating your data workloads in Google Cloud)
- Disaster recovery and fault tolerance (Google Documentation: What is a Disaster Recovery Plan?)
- Making decisions related to ACID (atomicity, consistency, isolation, and durability) compliance and availability
- Data validation
1.3 Designing for flexibility and portability. Considerations include
- Mapping current and future business requirements to the architecture
- Designing for data and application portability (e.g., multi-cloud and data residency requirements) (Google Documentation: Implement data residency and sovereignty requirements, Multicloud database management: Architectures, use cases, and best practices)
- Data staging, cataloging, and discovery (data governance) (Google Documentation: Data Catalog overview)
1.4 Designing data migrations. Considerations include:
- Analyzing current stakeholder needs, users, processes, and technologies and creating a plan to get to desired state
- Planning migration to Google Cloud (e.g., BigQuery Data Transfer Service, Database Migration Service, Transfer Appliance, Google Cloud networking, Datastream) (Google Documentation: Migrate to Google Cloud: Transfer your large datasets, Database Migration Service)
- Designing the migration validation strategy (Google Documentation: Migrate to Google Cloud: Best practices for validating a migration plan, About migration planning)
- Designing the project, dataset, and table architecture to ensure proper data governance (Google Documentation: Introduction to data governance in BigQuery, Create datasets)
Section 2: Ingesting and processing the data (25%)
2.1 Planning the data pipelines. Considerations include:
- Defining data sources and sinks (Google Documentation: Sources and sinks)
- Defining data transformation logic (Google Documentation: Introduction to data transformation)
- Networking fundamentals
- Data encryption (Google Documentation: Data encryption options)
2.2 Building the pipelines. Considerations include:
- Data cleansing
- Identifying the services (e.g., Dataflow, Apache Beam, Dataproc, Cloud Data Fusion, BigQuery, Pub/Sub, Apache Spark, Hadoop ecosystem, and Apache Kafka) (Google Documentation: Dataflow overview, Programming model for Apache Beam)
- Transformation:
- Batch (Google Documentation: Get started with Batch)
- Streaming (e.g., windowing, late arriving data)
- Language
- Ad hoc data ingestion (one-time or automated pipeline) (Google Documentation: Design Dataflow pipeline workflows)
- Data acquisition and import (Google Documentation: Exporting and Importing Entities)
- Integrating with new data sources (Google Documentation: Integrate your data sources with Data Catalog)
2.3 Deploying and operationalizing the pipelines. Considerations include:
- Job automation and orchestration (e.g., Cloud Composer and Workflows) (Google Documentation: Choose Workflows or Cloud Composer for service orchestration, Cloud Composer overview)
- CI/CD (Continuous Integration and Continuous Deployment)
Section 3: Storing the data (20%)
3.1 Selecting storage systems. Considerations include:
- Analyzing data access patterns (Google Documentation: Data analytics and pipelines overview)
- Choosing managed services (e.g., Bigtable, Cloud Spanner, Cloud SQL, Cloud Storage, Firestore, Memorystore) (Google Documentation: Google Cloud database options)
- Planning for storage costs and performance (Google Documentation: Optimize cost: Storage)
- Lifecycle management of data (Google Documentation: Options for controlling data lifecycles)
3.2 Planning for using a data warehouse. Considerations include:
- Designing the data model (Google Documentation: Data model)
- Deciding the degree of data normalization (Google Documentation: Normalization)
- Mapping business requirements
- Defining architecture to support data access patterns (Google Documentation: Data analytics design patterns)
3.3 Using a data lake. Considerations include
- Managing the lake (configuring data discovery, access, and cost controls) (Google Documentation: Manage a lake, Secure your lake)
- Processing data (Google Documentation: Data processing services)
- Monitoring the data lake (Google Documentation: What is a Data Lake?)
3.4 Designing for a data mesh. Considerations include:
- Building a data mesh based on requirements by using Google Cloud tools (e.g., Dataplex, Data Catalog, BigQuery, Cloud Storage) (Google Documentation: Build a data mesh, Build a modern, distributed Data Mesh with Google Cloud)
- Segmenting data for distributed team usage (Google Documentation: Network segmentation and connectivity for distributed applications in Cross-Cloud Network)
- Building a federated governance model for distributed data systems
Section 4: Preparing and using data for analysis (15%)
4.1 Preparing data for visualization. Considerations include:
- Connecting to tools
- Precalculating fields (Google Documentation: Introduction to materialized views)
- BigQuery materialized views (view logic) (Google Documentation: Create materialized views)
- Determining granularity of time data (Google Documentation: Filtering and aggregation: manipulating time series, Structure of Detailed data export)
- Troubleshooting poor performing queries (Google Documentation: Diagnose issues)
- Identity and Access Management (IAM) and Cloud Data Loss Prevention (Cloud DLP) (Google Documentation: IAM roles)
4.2 Sharing data. Considerations include:
- Defining rules to share data (Google Documentation: Secure data exchange with ingress and egress rules)
- Publishing datasets (Google Documentation: BigQuery public datasets)
- Publishing reports and visualizations
- Analytics Hub (Google Documentation: Introduction to Analytics Hub)
4.3 Exploring and analyzing data. Considerations include:
- Preparing data for feature engineering (training and serving machine learning models)
- Conducting data discovery (Google Documentation: Discover data)
Section 5: Maintaining and automating data workloads (18%)
5.1 Optimizing resources. Considerations include:
- Minimizing costs per required business need for data (Google Documentation: Migrate to Google Cloud: Minimize costs)
- Ensuring that enough resources are available for business-critical data processes (Google Documentation: Disaster recovery planning guide)
- Deciding between persistent or job-based data clusters (e.g., Dataproc) (Google Documentation: Dataproc overview)
5.2 Designing automation and repeatability. Considerations include:
- Creating directed acyclic graphs (DAGs) for Cloud Composer (Google Documentation: Write Airflow DAGs, Add and update DAGs)
- Scheduling jobs in a repeatable way (Google Documentation: Schedule and run a cron job)
5.3 Organizing workloads based on business requirements. Considerations include:
- Flex, on-demand, and flat rate slot pricing (index on flexibility or fixed capacity) (Google Documentation: Introduction to workload management, Introduction to legacy reservations)
- Interactive or batch query jobs (Google Documentation: Run a query)
5.4 Monitoring and troubleshooting processes. Considerations include:
- Observability of data processes (e.g., Cloud Monitoring, Cloud Logging, BigQuery admin panel) (Google Documentation: Observability in Google Cloud, Introduction to BigQuery monitoring)
- Monitoring planned usage
- Troubleshooting error messages, billing issues, and quotas (Google Documentation: Troubleshoot quota errors, Troubleshoot quota and limit errors)
- Manage workloads, such as jobs, queries, and compute capacity (reservations) (Google Documentation: Workload management using Reservations)
5.5 Maintaining awareness of failures and mitigating impact. Considerations include:
- Designing system for fault tolerance and managing restarts (Google Documentation: Designing resilient systems)
- Running jobs in multiple regions or zones (Google Documentation: Serve traffic from multiple regions, Regions and zones)
- Preparing for data corruption and missing data (Google Documentation: Verifying end-to-end data integrity)
- Data replication and failover (e.g., Cloud SQL, Redis clusters) (Google Documentation: High availability and replicas)
Google Cloud Professional Data Engineer Study Guide
Learning Resources for Google Cloud Certified Professional Data Engineer
For preparing for any certification or exam, it should be very clear if you know where to begin. The Google Cloud Certified Professional Data Engineer Difficulty is really high so you must follow a exam guide. To start the ideal preparation for the Google Cloud Certified Professional Data Engineer, the following details a few of the analytical steps that you should consider for developing an ideal schedule for your preparation.
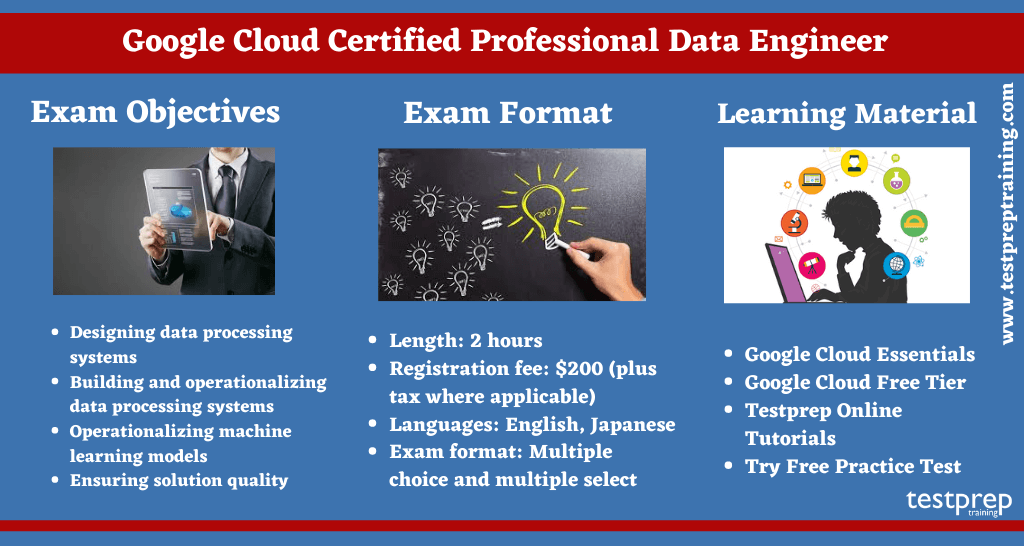
Google Official Website– First of all, you should visit the official website of Google because it offers the most reliable information about the exam. Google provides a study guide for each of its certifications and exams. The Google Cloud Certified Professional Data Engineer course outline is also present on the Google website. Moreover, the study guide provides all the reliable and most relevant information on a particular exam. It also includes the objectives and the basic details about the exam.
Evaluate yourself with Hands-on practice!– Since this particular exam tests technical skills related to the job profiles. Hence Hands-on experience is the best preparation for the exam. If after the training program candidates feel like having more experience or practice, we strongly suggest using the hands-on labs available on Qwiklabs. Also, they are available on the GCP free tier to grade up candidates’ knowledge and skills.
Google Learning Resources
Google offers the following Google Cloud Certified Professional Data Engineer Training program:
Google Cloud Free Tier– The Google Cloud Free Tier provides the candidate with free resources to study Google Cloud services. This becomes all the more enriching for a candidate if they are completely new to the platform and need to learn the basics. On the other side, if you’re a current customer looking to explore new solutions, the Google Cloud Free Tier is there for you.
Google Cloud Essentials– In this introductory-level quest, the candidate will get hands-on practice with Google Cloud’s fundamental tools and services. Google Cloud Essentials is the recommended first Quest for the Google Cloud learner. This provides the candidate with practical experience that they can apply to their first Google Cloud project. From writing Cloud Shell commands and marshaling their first virtual machine, to running applications on Kubernetes Engine or with load balancing. All this can be easily done with the help of Google Cloud Essential. Since it is the prime introduction to the platform’s basic features.
Additional Learning Resources – When it comes to certification exams like Google Cloud Certified Professional Data Engineer, the more the learning resources, the better will be the outcome. In the same vein, if the candidate requires more in-depth knowledge and wants to critically acknowledge their components of Google Cloud Platform. So, for that, we’re providing you two Quick links for additional resources.
- Google Cloud Platform Documentation
- Technical Guides
- Official Google Cloud Certified Professional Data Engineer Study Guide
Testprep Learning Resources
Testprep Online Tutorials– Google Cloud Certified Professional Data Engineer Online Tutorial boosts your knowledge, offering a thorough understanding of exam concepts. It covers exam details and policies, providing in-depth information about the examination. This helps you prepare effectively, strengthening your readiness. Learning through Online Tutorials is a valuable step in your preparation.
Try Practice Test– Google Cloud Certified Professional Data Engineer Practice Exams ensure that candidates are well-prepared. These tests help candidates identify their weak areas for improvement. With various practice tests available online, candidates can choose the ones that suit them. Testprep training also provides beneficial practice tests for those preparing for the exam.