Google Cloud Certified Professional Data Engineer
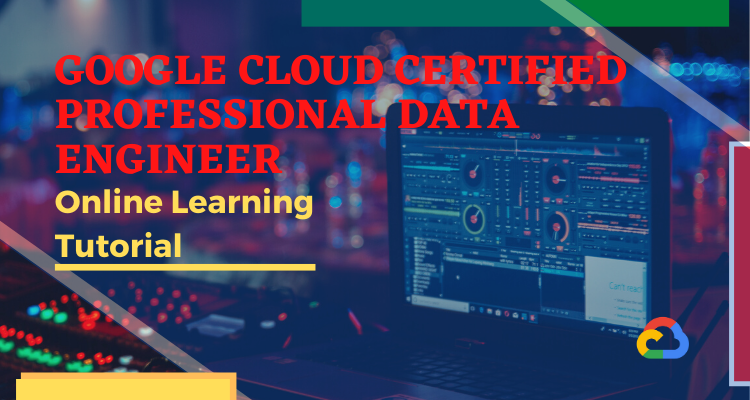
Data Engineers make it feasible for corporations to mesh in all the fancy advanced analytics and insight generation that data science offers. All this is done by creating trust and industry-wide access to accurate, reliable data at scale with sound data infrastructure and architecture. The Google Cloud Certified Professional Data Engineer helps you broaden your career prospects. Moreover, Google Cloud Certified Professional Data Engineer Salary is estimated to be is $132,900 USD. This certification will definitely help you make big in your professional life.
A Professional Data Engineer facilitates data-driven decision making by collecting, transforming, and publishing data. Data Engineer designs, operationalizes, secures and monitors data processing systems with a particular emphasis on security and compliance. Not to mention, the scalability and efficiency, reliability and fidelity, flexibility and portability. A Data Engineer leverages, deploys, and continuously train pre-existing machine learning models.
The Google Cloud Certified Professional Data Engineer exam assesses your ability to:
- Designing data processing systems
- Building and operationalizing data processing systems
- Operationalizing machine learning models
- Ensuring solution quality
Learning Objectives
Some of the skills required to become a successful Google Cloud Platform Data Engineer (GCP Data Engineer) are as follows:
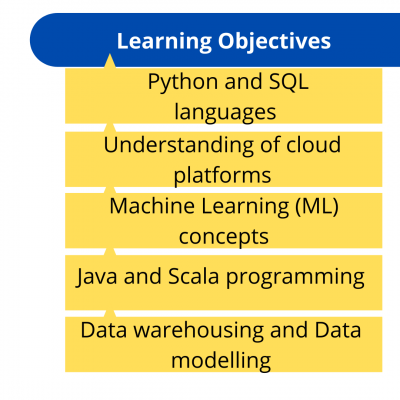
- Proficiency in Python and SQL languages
- Understanding of cloud platforms
- Knowledge of Machine Learning (ML) concepts
- Basic concepts of Java and Scala programming
- Knowledge of SQL and NoSQL databases
- Principles of data warehousing and data modelling
Prerequisites of the Exam
Prerequisities forms a very essential part of any exam. Regarding the Google Cloud Certified Professional Data Engineer requirements, the following are the requirements:
- Candidate should possess scalability and efficiency
- S/he should be able to design and monitor data processing systems with a particular emphasis on security
- Above all, a data engineer should be able to leverage and continuously train pre-existing machine learning models.
Exam Details
Before we proceed to the prerequisites for Google data engineer certification exam, we must know the requirement for the particular exam.
Google Data Engineer Certification exam comprises of 15 questions which are multiple select and multiple choice. You will be given 2 hours to complete the test and score 70% to get through the exam. Further, the exam is valid for 2 years and is available in two languages: English and Japanese. Above all, the exam costs $200 USD.
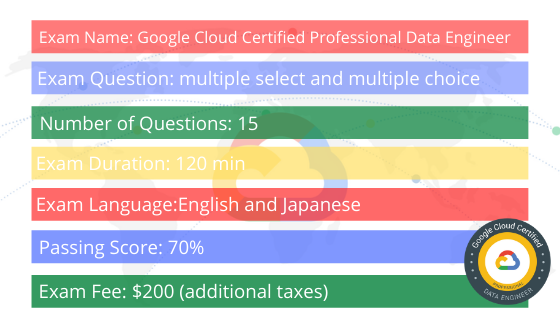
Course Outline: Google Cloud Professional Data Engineer
Take a glance at the topics that needed to be covered for the exam and you need to pay focus on:
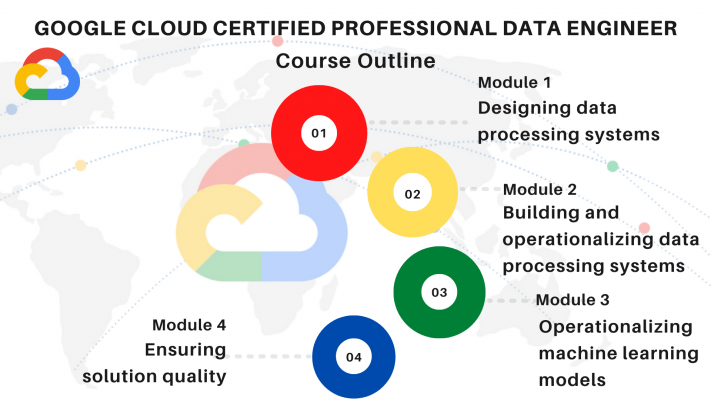
1. Designing data processing systems
1.1 Selecting the appropriate storage technologies.
- Mapping storage systems to business requirements (Google Documentation: Best practices for enterprise organizations)
- Data modeling (Google Documentation: Schema and data model, Data model)
- Tradeoffs involving latency, throughput, transactions (Google Documentation: Database consistency)
- Distributed systems (Google Documentation: Using clusters for large-scale technical computing in the cloud, choosing the right architecture for global data distribution)
- Schema design (Google Documentation: Designing your schema)
1.2 Designing data pipelines.
- Data publishing and visualization (e.g., BigQuery) (Google Documentation: Overview of Visual Profiling, Visualizing BigQuery data using Data Studio)
- Batch and streaming data (e.g., Cloud Dataflow, Cloud Dataproc, Apache Beam, Apache Spark and Hadoop ecosystem, Cloud Pub/Sub, Apache Kafka) (Google Documentation: Dataflow, Stream analytics)
- Online (interactive) vs. batch predictions (Google Documentation: Online versus batch prediction)
- Job automation and orchestration (e.g., Cloud Composer) (Google Documentation: Cloud Composer)
1.3 Designing a data processing solution.
- Choice of infrastructure
- System availability and fault tolerance (Google Documentation: Reliability, Overview of the high availability configuration)
- Use of distributed systems (Google Documentation: Using clusters for large-scale technical computing in the cloud, choosing the right architecture for global data distribution)
- Capacity planning (Google Documentation: Google Cloud Platform for Data Center Professionals: Compute)
- Hybrid cloud and edge computing (Google Documentation: Hybrid and multi-cloud architecture patterns)
- Architecture options (e.g., message brokers, message queues, middleware, service-oriented architecture, serverless functions) (Google Documentation: Pub/Sub)
- At least once, in-order, and exactly once, etc., event processing (Google Documentation: Exactly-once processing in Google Cloud Dataflow)
1.4 Migrating data warehousing and data processing.
- Awareness of current state and how to migrate a design to a future state (Google Documentation: Migration to Google Cloud: Assessing and discovering your workloads, Migration to Google Cloud: Getting started)
- Migrating from on-premises to cloud (Data Transfer Service, Transfer Appliance, Cloud Networking) (Google Documentation: CLOUD DATA TRANSFER)
- Validating a migration (Google Documentation: Migration to Google Cloud: Getting started)
2. Building and operationalizing data processing systems
2.1 Building and operationalizing storage systems.
- Effective use of managed services (Cloud Bigtable, Cloud Spanner, Cloud SQL, BigQuery, Cloud Storage, Cloud Datastore, Cloud Memorystore) (Google Documentation: Google Cloud Databases, Cloud Bigtable)
- Storage costs and performance (Google Documentation: Cloud Storage pricing, Best practices for Cloud Storage cost optimization)
- Lifecycle management of data (Google Documentation: Object Lifecycle Management)
2.2 Building and operationalizing pipelines.
- Data cleansing (Google Documentation: Cleanse Tasks)
- Batch and streaming (Google Documentation: Dataflow, Dataflow Under the Hood)
- Transformation (Google Documentation: Transform Basics)
- Data acquisition and import (Google Documentation: Best practices for importing and exporting data, CLOUD DATA TRANSFER)
- Integrating with new data sources (Google Documentation: Introduction to external data sources)
2.3 Building and operationalizing processing infrastructure. Considerations
- Provisioning resources (Google Documentation: Provisioning Overview, Infrastructure as code)
- Monitoring pipelines (Google Documentation: Using Monitoring for Dataflow pipelines, Using the Dataflow monitoring interface)
- Adjusting pipelines (Google Documentation: Updating an existing pipeline)
- Testing and quality control (Google Documentation: DevOps tech: Continuous testing)
3. Operationalizing machine learning models
3.1 Leveraging pre-built ML models as a service. Considerations
- ML APIs (e.g., Vision API, Speech API) (Google Documentation: Vision AI, Cloud Vision)
- Customizing ML APIs (e.g., AutoML Vision, Auto ML text) (Google Documentation: AutoML Vision)
- Conversational experiences (e.g., Dialogflow) (Google Documentation: Dialogflow)
3.2 Deploying an ML pipeline. Considerations
- Ingesting appropriate data (Google Documentation: Data lifecycle)
- Retraining of machine learning models (Cloud Machine Learning Engine, BigQuery ML, Kubeflow, Spark ML) (Google Documentation: Getting started with Kubeflow Pipelines, AI Platform)
- Continuous evaluation (Google Documentation: Continuous evaluation)
3.3 Choosing the appropriate training and serving infrastructure. Considerations
- Distributed vs. single machine (Google Documentation: Choosing the right architecture for global data distribution, Specifying machine types or scale tiers)
- Use of edge compute (Google Documentation: Google Cloud IoT)
- Hardware accelerators (e.g., GPU, TPU) (Google Documentation: Cloud Tensor Processing Units (TPUs))
3.4 Measuring, monitoring, and troubleshooting machine learning models. Considerations
- Machine learning terminology (e.g., features, labels, models, regression, classification, recommendation, supervised and unsupervised learning, evaluation metrics) (Google Documentation: Machine Learning Glossary, Introduction to BigQuery ML)
- Impact of dependencies of machine learning models (Google Documentation: Building a Serverless Machine Learning Model, Machine learning workflow)
- Common sources of error (e.g., assumptions about data) (Google Documentation: Common error guidance)
4. Ensuring solution quality
4.1 Designing for security and compliance. Considerations
- Identity and access management (e.g., Cloud IAM) (Google Documentation: Identity and Access Management)
- Data security (encryption, key management) (Google Documentation: Encryption at rest in Google Cloud)
- Ensuring privacy (e.g., Data Loss Prevention API) (Google Documentation: Cloud Data Loss Prevention (DLP) API)
- Legal compliance (e.g., Health Insurance Portability, and Accountability Act (HIPAA), Children’s Online Privacy Protection Act (COPPA), FedRAMP, General Data Protection Regulation (GDPR)) (Google Documentation: Google Cloud Security and Compliance, Google Cloud & the General Data Protection Regulation (GDPR))
4.2 Ensuring scalability and efficiency. Considerations
- Building and running test suites (Google Documentation: Community Tutorials, Deploying to Cloud Run)
- Pipeline monitoring (e.g., Stackdriver) (Google Documentation: Using Monitoring for Dataflow pipelines)
- Assessing, troubleshooting, and improving data representations and data processing infrastructure (Google Documentation: Data preprocessing for machine learning: options and recommendations)
- Resizing and autoscaling resources (Google Documentation: Autoscaling groups of instances)
4.3 Ensuring reliability and fidelity. Considerations
- Performing data preparation and quality control (e.g., Cloud Dataprep) (Google Documentation: Dataprep by Trifacta)
- Verification and monitoring (Google Documentation: Cloud Monitoring)
- Planning, executing, and stress testing data recovery (fault tolerance, rerunning failed jobs, performing retrospective re-analysis) (Google Documentation: Disaster recovery planning guide)
- Choosing between ACID, idempotent, eventually consistent requirements (Google Documentation: Balancing Strong and Eventual Consistency with Datastore)
4.4 Ensuring flexibility and portability. Considerations
- Mapping to current and future business requirements (Google Documentation: Best practices for enterprise organizations)
- Designing for data and application portability (e.g., multi-cloud, data residency requirements) (Google Documentation: Hybrid and multi-cloud patterns and practices)
- Data staging, cataloguing, and discovery (Google Documentation: Data Catalog overview)
The whole Certification Process
As mentioned above, the exam is online Proctored examination.
Exam Delivery Method:
- Take the online-proctored exam from a remote location, review the online testing requirements.
- Take the onsite-proctored exam at a testing center, Locate a test center near you.
Book/Schedule the exam
If you are determined enough to go for this exam and become a certified Google Data Engineer, then it’s time to register for the exam and go-ahead for the preparation. Following are the steps to apply for the exam:
To book the exam, you can go to the Official Google Cloud website.
- You will need a Web assessor account. You are supposed to create a one in order to register yourself for the exam. To create, click here
- Create the account with your personal email address and not your work address.
- Check the catalogue and register for the exam you want to apply for.
- Choose the exam centre i.e. Kryterion Testing Centre.
- When you register for an exam, you will need to schedule an exam time at a Kryterion testing centre that is convenient for you. You can locate the nearest testing centre here.
Retake the Exam
For instance, if you don’t pass the certification exam, you can take it again after 14 days.
Similarly, if you don’t pass the second time, you must wait 60 days.
Further, if you don’t pass the third attempt, you’ll have to wait a year before trying again. Above all, payment is required each time you take an exam.
Note: Trying to sidestep the retake policy by registering under a different name is a violation of the exam terms and conditions and will result in a denied or revoked certification.
Maintaining the Certification
It is highly important to note that all Google cloud certifications are valid for two years from the date certified. Therefore, candidates must recertify their certificates in order to maintain their certification status and certificate number.
Exam Day
After crossing all the hurdles, comes your exam day. All your hard work is going to take shape. In other words, you are going to get your output in your hand. In order to receive one, you should remember the following points:
- Firstly, you should arrive before the arrival time at the exam centre with valid identity proof (a voter ID card which differs depending upon the location and country).
- Secondly, you can just contact the customer care of the testing centre to take information about the perquisites of the exam or check the official certification page.
- Thirdly, you will be given a locker where you can keep your belongings at the test centre.
Exam Policies
Google Cloud Certification provides exam policies to support the candidates by providing every detail related to the certification program. However, candidates during studying for the Google Cloud Professional Data Engineer exam should first go through and understand the Google Cloud Certification exam policies. On this page, the candidates will get information about after the exam or before exam procedures. This includes the exam retaking process, rules to be followed during the exam time, and other information about the exams and its testing centres.
While preparing for the Google Cloud Professional Data Engineer exam you will be solely responsible for understanding and complying with Google Cloud Professional Data Engineer exam policies, together with the specified exam delivery provider’s policies and procedures.
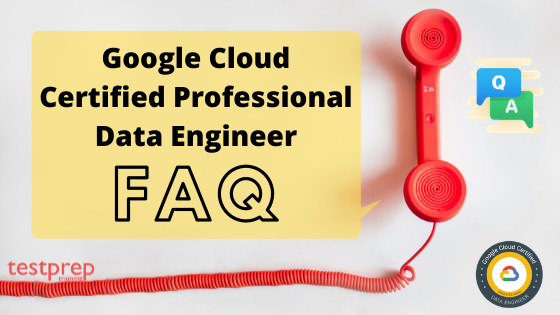
For more queries, visit the Google Cloud Certified Professional Data Engineer FAQ.
Preparation Resources to become a Google Cloud Certified Professional Data Engineer
As you commence your preparation for GCP Cloud Developer certification exam, there are some common-yet-powerful methods that are beneficial in your preparation. There are so many candidates who prepare for certification by studying a book and later are disappointed if they can’t qualify the exam. However, the reality is much different than the expectation. Just acknowledging the source information is only a small part of the preparation guide.
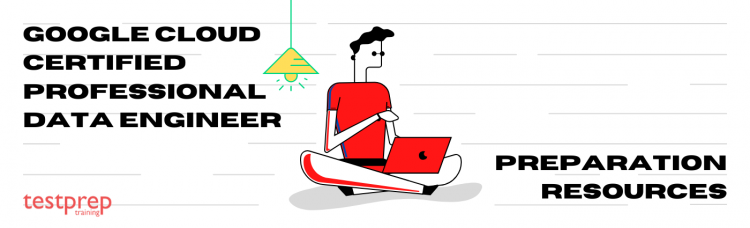
Review the Exam Guide
The exam guide has a complete list of topics and domains that are included in the exam. So, review the exam guide to determine if your skills align with the topics on the exam. This will allow you to have a better understanding of the Google Cloud Certified Professional Data Engineer exam.
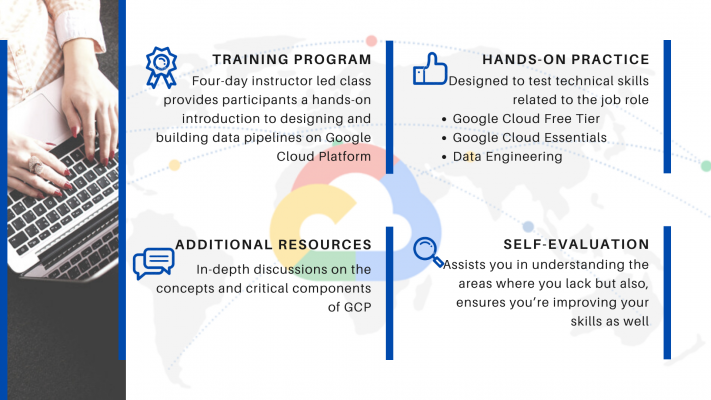
Get started with Training Program
When it comes to certification exams, there’s nothing better than the Google Cloud Certified Professional Data Engineer Training programs. These offer the candidates with such deep knowledge and insights of the Google Cloud Platform.
Data Engineering on Google Cloud Platform
This four-day instructor-led class provides participants with a hands-on introduction to designing and building data pipelines on Google Cloud Platform. With a combination of presentations, demos, and hands-on labs, candidates learn the process of designing a data system. Not to mention, they also learn and build end-to-end data pipelines, analyze data and derive insights. This particular course entails everything structured, unstructured, and streaming data.
Don’t underestimate the power of Hands-on practice!
Since this particular exam tests technical skills related to the job profiles. Hence Hands-on experience is the best preparation for the exam. If after training program candidates feel like having more experience or practise, we strongly suggest using the hands-on labs available on Qwiklabs. Also, they are available on the GCP free tier to grade up candidates knowledge and skills.
Google Cloud Free Tier
The Google Cloud Free Tier provides the candidate with free resources to study Google Cloud services. This becomes all the more enriching for a candidate if they are completely new to the platform and need to learn the basics. On the other hand, if suppose you’re an established customer and want to experiment with new solutions, the Google Cloud Free Tier has got you covered.
Google Cloud Essentials
In this introductory-level quest, the candidate will get hands-on practice with Google Cloud’s fundamental tools and services. Google Cloud Essentials is the recommended first Quest for the Google Cloud learner. This provides the candidate with practical experience that they can apply to their first Google Cloud project. From writing Cloud Shell commands and marshaling their first virtual machine, to running applications on Kubernetes Engine or with load balancing. All this can be easily done with the help of Google Cloud Essential. Since it is the prime introduction to the platform’s basic features.
Data Engineering
This advanced-level quest is unparalleled amongst the other Qwiklabs offerings. The labs are curated to provide the IT professionals hands-on practice with topics and services that appear in the Google Cloud Certified Professional Data Engineer Certification. From Big Query to Dataprep, to Cloud Composer & Tensorflow, this quest is composed of specific labs that will put your GCP data engineering knowledge to the test. Not to mention, this will increase candidates’ skills and abilities, so they won’t require other preparation. As the exam is quite challenging. Therefore, external studying, practice, or background in cloud data engineering is urged.
Additional resources for the Professional Data Engineer in you
When it comes to certification exams like Google Cloud Certified Professional Data Engineer, the more the learning resources, the better will be the outcome. In the same vein, if candidate requires more in-depth knowledge and wants to critically acknowledge their components of Google Cloud Platform. So, for that, we’re providing you two Quick links for additional resources.
- Google Cloud Platform Documentation
- Official Google Cloud Certified Professional Data Engineer Study Guide
- Technical Guides
Self-Evaluation makes you better
And, finally, it’s time for self-evaluation. Take it from us, Self Evaluation is the last step of your success. Therefore Google Cloud Certified Professional Data Engineer Practice Exams are all you need. The more you’re going to practice, it’s better for you.
Not only does it assists you in understanding the areas where you lack but also, ensures you’re improving your skills as well. So, keep on practicing as many practice tests as much you can. FOR MORE PRACTICE TEST, CLICK HERE!
A certification is just a test away. So, prepare with the online Learning tutorial and advanced learning resources to become a Google Cloud Certified Professional Data Engineer Now!
Google Cloud Professional Data Engineer Learning Resources
The Professional Data Engineer exam enables data-driven decision making by collecting, transforming, and visualizing data. The sole objective of a Google Cloud Certified – Professional Data Engineer is to design, build, maintain, and troubleshoot data processing systems with a particular emphasis on the security, reliability, fault-tolerance, scalability, fidelity, and efficiency of the systems.
Table of Contents
Cloud Basics
Storage Models
Schema Design
- What is Schema Design
- Relational Schema Design
- Non-relational Schema Design in Cloud Bigtable
- Table design
- Bigtable Overview
- Cloud Bigtable architecture
- Data Organization
- Load balancing
- Columns
- Supported data types
- Empty cells
- Column qualifiers
- Compactions
- Mutations and deletions
- Data compression
- Data durability
- Security
- Instance Configuration
- Storage types
- Data Management
- cbt tool
- Best Practices
- Cloud Dataflow Overview
- Key Concepts
- Pipeline Design
- Pipeline Lifecycle, creation and Transform
- Cloud Dataflow templates
- Streaming Pipeline
- Best Practices
- BigQuery Overview
- Interacting with BigQuery
- Loading Data
- Exporting Data
- Optimize for Performance and Cost
- Queries
- BigQuery Logging and Monitoring
- BigQuery Best Practices
Machine Learning
Google AI Platform (Formerly Cloud ML Engine)
Pre-trained Machine Learning API’s
Security and Compliance
- Google Security Culture
- Operational Security
- Technology with Security at Its Core
- Independent Third-Party Certifications
- Regulatory compliance
- General Data Protection Regulation (GDPR)
- The Health Insurance Portability and Accountability Act of 1996 (HIPAA)
- Cloud Data Loss Prevention (DLP)
- Encryption Basics
- Cloud Key Management Service