The best way to stand out in this competitive job sector is to hold a certification with advanced level skills. And, as we know that AWS cloud computing services are one of the fastest-growing sectors even in 2020 which is considered to be amongst the most difficult years of all times. Whilst, maintaining its norms, AWS focuses on upgrading its certification level by staying up to date with the latest technologies. However, in 2020, when the world was dealing with COVID-19, AWS was working on updates and improvements in its exam to provide more visibility for candidates to earn the certification.
As a result, in March 2021, a new version of the AWS Certified Big Data – Specialty (BDS-C00) exam was launched which is AWS Certified Data Analytics – Specialty (DAS-C01) Exam. AWS DAS-C01 exam will help in achieving candidates an industry-recognized credential from AWS with validating skills in AWS data lakes and analytics services.
n this blog, we will learn everything about the AWS Certified Data Analytics – Specialty (DAS-C01) Exam, starting from the exam overview and then about the useful resources that will lead you to understand it more accurately.
AWS Certified Data Analytics Specialty Exam Overview
With building credibility and confidence by highlighting the abilities to design, build, secure, and maintain analytics solutions on AWS, AWS Certified Data Analytics – Specialty (DAS-C01) Exam is key to have a good career. However, this exam is best suitable if you are in the focused role of data analytics. As the exam measures your understanding of using AWS services for designing, building, maintaining, and securing analytics solutions for providing insight from data.
Further, the exam validates ability in:
- Firstly, defining the concepts of AWS data analytics services and understanding how they integrate with each other.
- Secondly, describing the process of AWS data analytics services and how it fits in the data lifecycle of collection, storage, processing, and visualization.
For AWS Certified Data Analytics – Specialty (DAS-C01) Exam, AWS provides knowledge requirements that may be recommended to gain before taking this exam. So, let’s take a look at it.
Recommended AWS Knowledge: DAS-C01 Exam
For AWS Certified Data Analytics – Specialty (DAS-C01) Exam,
- Firstly, five or more years of experience with common data analytics technologies
- Secondly, should have at least two years of hands-on experience working on AWS
- Lastly, knowledge and experience of working with AWS services for designing, building, securing, and maintaining analytics solutions.
As we all know that this certification was previously known as AWS Certified Big Data – Specialty. However, the certifications earned with Big Data are still active for three years from the date they are earned.
AWS Exam Details
For the AWS Specialty exam, it requires more information to understand the pattern before starting your preparation. So, below we will cover the exam details, procedures and course outline to create a clarity.
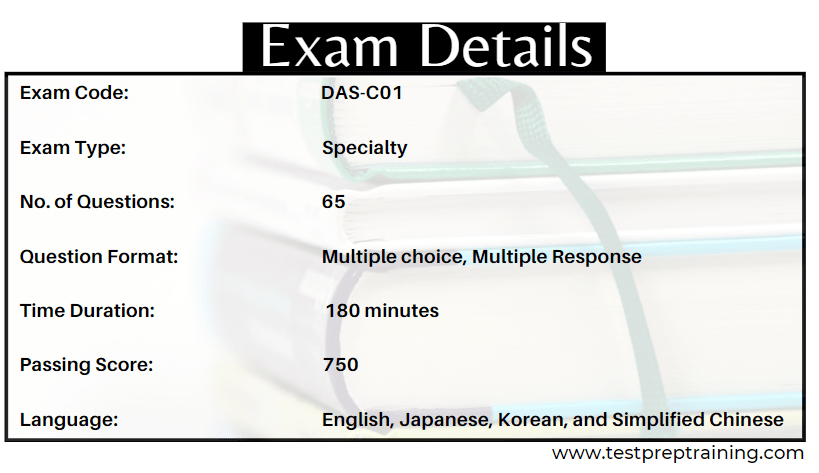
- AWS Certified Data Analytics – Specialty (DAS-C01) Exam consists of 65 questions that will be of either multiple choice or multiple response types.
- Secondly, to complete the exam, there will be a time limit of 180 minutes.
- Thirdly, this exam comes with two delivery methods:
- Testing center
- Online proctored exam
- Next, this exam will cost you 300 USD (Practice exam: 40 USD).
- Lastly, the exam can be taken in English, Japanese, Korean, and Simplified Chinese Language.
Before moving to the next section,take a look at some exam related information that will be useful during the exam.
Important Things you should know
- Firstly, there are two types of questions on the exam:
- Multiple choice. It has one correct response and three incorrect responses.
- Multiple responses. It has two or more correct responses out of five or more options.
- Secondly, the AWS Certified Data Analytics – Specialty (DAS-C01) exam is a pass or fail the exam. However, the results for the exam score are reported ranges from 100–1,000. And, the minimum passing score for this exam is 750.
- Lastly, the compensatory scoring model states that you do not need to “pass” the individual sections, only the overall exam. That is to say, each section of the examination has a specific weighting.
Exam Signing up benefits
AWS Certification helps individuals in building skills and knowledge by validating cloud expertise with industry-recognized certification. And, also for organizations in identifying skilled professionals for leading cloud initiatives using AWS. So, you can start preparing by signing up for AWS that will help you in:
- Firstly, scheduling and managing exams
- Secondly, viewing your certification history
- Thirdly, accessing your digital badges
- Next, for taking sample tests
- Lastly, viewing your certification benefits
Moving onto the important section that is exam content.
AWS Exam Content Outline
For AWS Certified Data Analytics – Specialty (DAS-C01) Exam, an exam guide is provided that includes the weightings, test domains, and objectives. The topics provided in this outline consists of sections that will make you understand about the topic more accurately. The topics include:
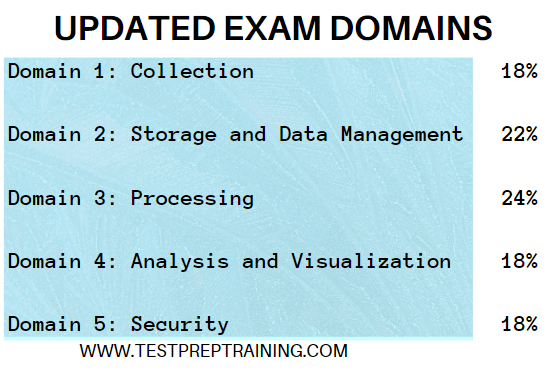
Domain 1: Collection
1.1 Determine the operational characteristics of the collection system
- Evaluate that the data loss is within tolerance limits in the event of failures (AWS Documentation: Fault tolerance, Failure Management)
- Evaluate costs associated with data acquisition, transfer, and provisioning from various sources into the collection system (e.g., networking, bandwidth, ETL/data migration costs) (AWS Documentation: Cloud Data Migration, Plan for Data Transfer, Amazon EC2 FAQs)
- Assess the failure scenarios that the collection system may undergo, and take remediation actions based on impact (AWS Documentation: Remediating Noncompliant AWS Resources, CIS AWS Foundations Benchmark controls, Failure Management)
- Determine data persistence at various points of data capture (AWS Documentation: Capture data)
- Identify the latency characteristics of the collection system (AWS Documentation: I/O characteristics and monitoring, Amazon CloudWatch concepts)
1.2 Select a collection system that handles the frequency, volume, and the source of data
- Describe and characterize the volume and flow characteristics of incoming data (streaming, transactional, batch) (AWS Documentation: Characteristics, streaming data)
- Match flow characteristics of data to potential solutions
- Assess the tradeoffs between various ingestion services taking into account scalability, cost, fault tolerance, latency, etc. (AWS Documentation: Amazon EMR FAQs, Data ingestion methods)
- Explain the throughput capability of a variety of different types of data collection and identify bottlenecks (AWS Documentation: Caching Overview, I/O characteristics and monitoring)
- Choose a collection solution that satisfies connectivity constraints of the source data system
1.3 Select a collection system that addresses the key properties of data, such as order, format, and compression
- Describe how to capture data changes at the source (AWS Documentation: Capture changes from Amazon DocumentDB, Creating tasks for ongoing replication using AWS DMS, Using change data capture)
- Discuss data structure and format, compression applied, and encryption requirements (AWS Documentation: Compression encodings, Athena compression support)
- Distinguish the impact of out-of-order delivery of data, duplicate delivery of data, and the tradeoffs between at-most-once, exactly-once, and at-least-once processing (AWS Documentation: Amazon SQS FIFO (First-In-First-Out) queues, Amazon Simple Queue Service)
- Describe how to transform and filter data during the collection process (AWS Documentation: Transform Data, Filter class)
Domain 2: Storage and Data Management
2.1 Determine the operational characteristics of the storage solution for analytics
- Determine the appropriate storage service(s) on the basis of cost vs. performance (AWS Documentation: Amazon S3 pricing, Storage Architecture Selection)
- Understand the durability, reliability, and latency characteristics of the storage solution based on requirements (AWS Documentation: Storage, Selection)
- Determine the requirements of a system for strong vs. eventual consistency of the storage system (AWS Documentation: Amazon S3 Strong Consistency, Consistency Model)
- Determine the appropriate storage solution to address data freshness requirements (AWS Documentation: Storage, Storage Architecture Selection)
2.2 Determine data access and retrieval patterns
- Determine the appropriate storage solution based on update patterns (e.g., bulk, transactional, micro batching) (AWS Documentation: select your storage solution, Performing large-scale batch operations, Batch data processing)
- Determine the appropriate storage solution based on access patterns (e.g., sequential vs. random access, continuous usage vs.ad hoc) (AWS Documentation: optimizing Amazon S3 performance, Amazon S3 FAQs)
- Determine the appropriate storage solution to address change characteristics of data (appendonly changes vs. updates)
- Determine the appropriate storage solution for long-term storage vs. transient storage (AWS Documentation: Storage, Using Amazon S3 storage classes)
- Determine the appropriate storage solution for structured vs. semi-structured data (AWS Documentation: Ingesting and querying semistructured data in Amazon Redshift, Storage Best Practices for Data and Analytics Applications)
- Determine the appropriate storage solution to address query latency requirements (AWS Documentation: In-place querying, Performance Guidelines for Amazon S3, Storage Architecture Selection)
2.3 Select appropriate data layout, schema, structure, and format
- Determine appropriate mechanisms to address schema evolution requirements (AWS Documentation: Handling schema updates, Best practices for securing sensitive data in AWS data stores)
- Select the storage format for the task (AWS Documentation: Task definition parameters, Specifying task settings for AWS Database Migration Service tasks)
- Select the compression/encoding strategies for the chosen storage format (AWS Documentation: Choosing compression encodings for the CUSTOMER table, Compression encodings)
- Select the data sorting and distribution strategies and the storage layout for efficient data access (AWS Documentation: Best practices for using sort keys to organize data, Working with data distribution styles)
- Explain the cost and performance implications of different data distributions, layouts, and formats (e.g., size and number of files) (AWS Documentation: optimizing Amazon S3 performance)
- Implement data formatting and partitioning schemes for data-optimized analysis (AWS Documentation: Partitioning data in Athena, Partitions and data distribution)
2.4 Define data lifecycle based on usage patterns and business requirements
- Determine the strategy to address data lifecycle requirements (AWS Documentation: Amazon Data Lifecycle Manager)
- Apply the lifecycle and data retention policies to different storage solutions (AWS Documentation: Setting lifecycle configuration on a bucket, Managing your storage lifecycle)
2.5 Determine the appropriate system for cataloging data and managing metadata
- Evaluate mechanisms for discovery of new and updated data sources (AWS Documentation: Discovering on-premises resources using AWS discovery tools)
- Evaluate mechanisms for creating and updating data catalogs and metadata (AWS Documentation: Catalog and search, Data cataloging)
- Explain mechanisms for searching and retrieving data catalogs and metadata (AWS Documentation: Understanding tables, databases, and the Data Catalog)
- Explain mechanisms for tagging and classifying data (AWS Documentation: Data Classification, Data classification overview)
Domain 3: Processing
3.1 Determine appropriate data processing solution requirements
- Understand data preparation and usage requirements (AWS Documentation: Data Preparation, Preparing data in Amazon QuickSight)
- Understand different types of data sources and targets (AWS Documentation: Targets for data migration, Sources for data migration)
- Evaluate performance and orchestration needs (AWS Documentation: Performance Efficiency)
- Evaluate appropriate services for cost, scalability, and availability (AWS Documentation: High availability and scalability on AWS)
3.2 Design a solution for transforming and preparing data for analysis
- Apply appropriate ETL/ELT techniques for batch and real-time workloads (AWS Documentation: ETL and ELT design patterns for lake house architecture)
- Implement failover, scaling, and replication mechanisms (AWS Documentation: Disaster recovery options in the cloud, Working with read replicas)
- Implement techniques to address concurrency needs (AWS Documentation: Managing Lambda reserved concurrency, Managing Lambda provisioned concurrency)
- Implement techniques to improve cost-optimization efficiencies (AWS Documentation: Cost Optimization)
- Apply orchestration workflows (AWS Documentation: AWS Step Functions)
- Aggregate and enrich data for downstream consumption (AWS Documentation: Joining and Enriching Streaming Data on Amazon Kinesis, Designing a High-volume Streaming Data Ingestion Platform Natively on AWS)
3.3 Automate and operationalize data processing solutions
- Implement automated techniques for repeatable workflows
- Apply methods to identify and recover from processing failures (AWS Documentation: Failure Management, Recover your instance)
- Deploy logging and monitoring solutions to enable auditing and traceability (AWS Documentation: Enable Auditing and Traceability)
Domain 4: Analysis and Visualization
4.1 Determine the operational characteristics of the analysis and visualization solution
- Determine costs associated with analysis and visualization (AWS Documentation: Analyzing your costs with AWS Cost Explorer)
- Determine scalability associated with analysis (AWS Documentation: Predictive scaling for Amazon EC2 Auto Scaling)
- Determine failover recovery and fault tolerance within the RPO/RTO (AWS Documentation: Plan for Disaster Recovery (DR))
- Determine the availability characteristics of an analysis tool (AWS Documentation: Analytics)
- Evaluate dynamic, interactive, and static presentations of data (AWS Documentation: Data Visualization, Use static and dynamic device hierarchies)
- Translate performance requirements to an appropriate visualization approach (pre-compute and consume static data vs. consume dynamic data)
4.2 Select the appropriate data analysis solution for a given scenario
- Evaluate and compare analysis solutions (AWS Documentation: Evaluating a solution version with metrics)
- Select the right type of analysis based on the customer use case (streaming, interactive, collaborative, operational)
4.3 Select the appropriate data visualization solution for a given scenario
- Evaluate output capabilities for a given analysis solution (metrics, KPIs, tabular, API) (AWS Documentation: Using KPIs, Using Amazon CloudWatch metrics)
- Choose the appropriate method for data delivery (e.g., web, mobile, email, collaborative notebooks) (AWS Documentation: Amazon SageMaker Studio Notebooks architecture, Ensure efficient compute resources on Amazon SageMaker)
- Choose and define the appropriate data refresh schedule (AWS Documentation: Refreshing SPICE data, Refreshing data in Amazon QuickSight)
- Choose appropriate tools for different data freshness requirements (e.g., Amazon Elasticsearch Service vs. Amazon QuickSight vs. Amazon EMR notebooks) (AWS Documentation: Amazon EMR, Choosing the hardware for your Amazon EMR cluster, Build an automatic data profiling and reporting solution)
- Understand the capabilities of visualization tools for interactive use cases (e.g., drill down, drill through, and pivot) (AWS Documentation: Adding drill-downs to visual data in Amazon QuickSight, Using pivot tables)
- Implement the appropriate data access mechanism (e.g., in memory vs. direct access) (AWS Documentation: Security Best Practices for Amazon S3, Identity and access management in Amazon S3)
- Implement an integrated solution from multiple heterogeneous data sources (AWS Documentation: Data Sources and Ingestion)
Domain 5: Security
5.1 Select appropriate authentication and authorization mechanisms
- Implement appropriate authentication methods (e.g., federated access, SSO, IAM) (AWS Documentation: Identity and access management for IAM Identity Center)
- Implement appropriate authorization methods (e.g., policies, ACL, table/column level permissions) (AWS Documentation: Managing access permissions for AWS Glue resources, Policies and permissions in IAM)
- Implement appropriate access control mechanisms (e.g., security groups, role-based control) (AWS Documentation: Implement access control mechanisms)
5.2 Apply data protection and encryption techniques
- Determine data encryption and masking needs (AWS Documentation: Protecting Data at Rest, Protecting data using client-side encryption)
- Apply different encryption approaches (server-side encryption, client-side encryption, AWS KMS, AWS CloudHSM) (AWS Documentation: AWS Key Management Service FAQs, Protecting data using server-side encryption, Cryptography concepts)
- Implement at-rest and in-transit encryption mechanisms (AWS Documentation: Encrypting Data-at-Rest and -in-Transit)
- Implement data obfuscation and masking techniques (AWS Documentation: Data masking using AWS DMS, Create a secure data lake by masking)
- Apply basic principles of key rotation and secrets management (AWS Documentation: Rotate AWS Secrets Manager secrets)
5.3 Apply data governance and compliance controls
- Determine data governance and compliance requirements (AWS Documentation: Management and Governance)
- Understand and configure access and audit logging across data analytics services (AWS Documentation: Monitoring audit logs in Amazon OpenSearch Service)
- Implement appropriate controls to meet compliance requirements (AWS Documentation: Security and compliance)
We have almost covered all the essential details related to the AWS Certified Data Analytics – Specialty (DAS-C01) Exam. Now, it’s time to talk about the study resources and the training methods that will help you in exam preparation.
Before that take a quick look at the benefits of the AWS certifications.
AWS Certification Benefits
- Firstly, AWS gives chance to learn from AWS experts and get yourself advanced. It provides flexibility by offering candidates to choose what to learn, and when and how to start.
- Secondly, this helps in increasing the credibility by providing direct assistance from AWS experts with having domain experience and access to the latest AWS Cloud products, services, and teaching methods.
- Thirdly, it helps in improvising solutions to meet your goals.
- Lastly, AWS offers virtual training to complement digital courses.
AWS Certified Data Analytics Specialty Preparation Guide
Before starting preparing for the exam it is important to have hands-on experience on the skills mentioned for the exam. Moreover, you should be having the best study resources and exam guide to cover everything in one place. Below we will talk about some of the essential training resources and methods useful for this exam.
AWS Exam guide
For every certification, AWS provides an exam guide that contains the content outline, exam overview, and the target audience for the certification exam. Before starting preparing for the exam, it is important to go through this guide to understand the knowledge requirement and other major details for the exam.
AWS Training
AWS helps in building your technical skills by providing recommended courses according to the exam. For AWS Certified Data Analytics – Specialty (DAS-C01) Exam the course include:
Data Analytics Fundamentals
In this course, you will understand and learn to plan data analysis solutions and the data analytic processes. This course provides five key factors indicating the need for specific AWS services to collect, process, analyze, and present data. Further, this course has objectives, some of them include:
- Firstly, identifying the characteristics of data analysis solutions and the characteristics indicating a solution.
- Secondly, defining types of data including structured, semistructured, and unstructured data.
- Thirdly, defining data storage types such as data lakes, AWS Lake Formation, data warehouses, and the Amazon Simple Storage Service (Amazon S3).
- Next, analyzing the characteristics of and differences in batch and stream processing.
- Then, defining how Amazon Kinesis is used to process streaming data.
- Lastly, analyzing the characteristics of different storage systems for source data and the differences of row-based and columnar data storage methods.
Big Data on AWS
Big Data on AWS provides knowledge of cloud-based big data solutions such as Amazon Elastic MapReduce (EMR), Amazon Redshift, and the rest of the AWS big data platform. However, in this course, you will learn the concept of using Amazon EMR In order to process data using the broad ecosystem of Hadoop tools like Hive and Hue. This will also help in:
- learning the process of creating big data environments
- working with services such as Amazon DynamoDB, Amazon Redshift, Amazon QuickSight, and Amazon Kinesis
- leveraging best practices for designing big data environments for security and cost-effectiveness.
Exam Readiness training by AWS
Exam Readiness training focuses on teaching the process of interpreting exam questions and allocating your study time. However, for this exam exam readiness provides training course that is,
Exam Readiness: AWS Certified Data Analytics – Specialty
This course helps you in your exam preparation by exploring the exam’s topic areas and familiarizing you with the questions format and exam approach. In this course, you will learn about:
- Firstly, navigating the logistics of the examination process
- Secondly, understanding the exam structure and question types
- Thirdly, identifying how questions relate to AWS data analytics concepts
- Next, interpret the concepts being tested by exam questions
- Lastly, developing a personalized study plan to prepare for the exam
Practice Tests
Taking a practice test is a great way to create a study strategy and to learn about your weak areas. Moreover, practice tests help you to have the best possible revision, and taking sample tests for the AWS Certified Data Analytics – Specialty (DAS-C01) Exam, will help in learning and understanding the pattern of the questions so that you don’t face any problem during the exam.
Final Words
By passing the new AWS Certified Data Analytics – Specialty (DAS-C01) Exam, you have open doors to many big opportunities and to achieve your goal. By holding an AWS Certified Data Analytics – Specialty credential, you will earn digital badges from AWS that you can use to display your abilities and even share your real-time achievement with employers and on social media. So, this new exam can provide you a source of benefits that can end up in having a good career. Further, you can start preparing for the exam using the list of training methods provided in this article.