Salesforce Einstein Analytics and Discovery Consultant Interview Questions
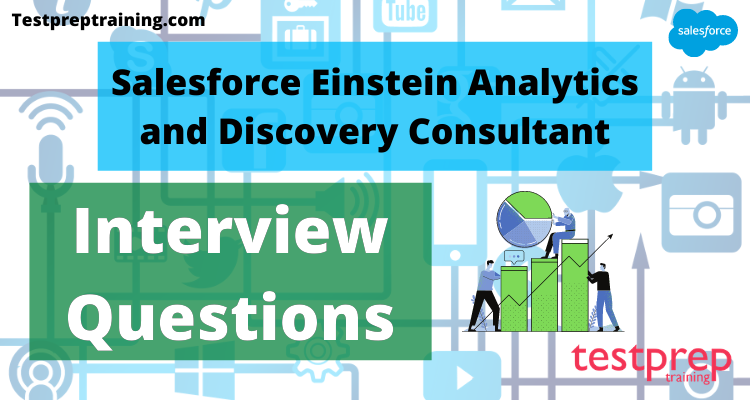
The Salesforce Einstein Analytics and Discovery Consultant exam is intended for all those who have the knowledge, skills, and experience with data ingestion processes, security and access implementations, and dashboard creation. This exam also checks your fundamental knowledge and skills to design, build, and support apps, datasets, dashboards and stories in Einstein Analytics and Discovery.
Although this certification is in high demand but cracking the exam isn’t easy. You need to pass its interview which requires in-depth knowledge of all the technical concepts. To solve this, we present you with Salesforce Einstein Analytics and Discovery Consultant Interview Questions which would give you an idea about what types and patterns of questions could be expected.
Advanced Interview Questions
What is Salesforce Einstein Analytics and how does it differ from traditional Business Intelligence tools?
Salesforce Einstein Analytics is a cloud-based business intelligence and analytics platform designed specifically for Salesforce users. It uses artificial intelligence and machine learning to analyze vast amounts of data and provide actionable insights to users in real-time. It provides a self-service analytics platform for businesses to gain deep insights into customer behavior, sales performance, marketing effectiveness, and other business metrics.
Salesforce Einstein Analytics differs from traditional business intelligence tools in several key ways:
- AI-powered Insights: Einstein Analytics uses machine learning algorithms to automatically identify patterns, trends, and anomalies in data. This makes it possible to uncover insights that would not be immediately obvious with traditional business intelligence tools.
- User-friendly Interface: Einstein Analytics is designed to be easy to use and accessible to business users without the need for technical skills or expertise. It provides a simple drag-and-drop interface, so users can quickly create dashboards and reports without writing any code.
- Real-time Data: Einstein Analytics provides real-time insights by leveraging Salesforce’s cloud-based data storage and analytics platform. This means that users can get up-to-date information on key metrics and make decisions based on the latest data.
- Customization: Einstein Analytics provides a customizable platform, allowing users to create tailored dashboards, reports, and visualizations that meet their specific needs. This makes it possible to align data insights with business goals and priorities.
- Integration with Salesforce: Einstein Analytics is fully integrated with Salesforce, making it possible to easily connect data from different Salesforce objects and perform cross-object analysis. This enables users to gain a more comprehensive view of customer and business data.
Overall, Salesforce Einstein Analytics provides a cutting-edge, AI-powered analytics platform that is designed to meet the needs of modern businesses. It provides real-time insights, a user-friendly interface, and the ability to customize and integrate with other Salesforce products.
How does Einstein Analytics integrate with Salesforce CRM?
Einstein Analytics is a business intelligence platform that is built on top of Salesforce CRM. It provides advanced data analytics, data visualization, and dashboards to help businesses make data-driven decisions. The integration between Einstein Analytics and Salesforce CRM enables businesses to access and analyze data from within the Salesforce CRM platform.
The integration between the two platforms is based on the Salesforce Connect technology, which allows Einstein Analytics to connect to Salesforce data sources and retrieve data from them. The data is then transformed, loaded, and stored in the Einstein Analytics data platform, where it can be analyzed and visualized.
Once the data is in the Einstein Analytics data platform, it can be used to create dashboards, reports, and visualizations that provide insights into business performance and trends. The dashboards and reports can be customized and tailored to the specific needs of the business and can be easily shared with other Salesforce users.
Additionally, Einstein Analytics provides advanced analytics capabilities, such as predictive analytics and machine learning, which can be used to identify trends and patterns in the data, forecast future outcomes, and make data-driven decisions. The results of these analytics can be displayed in the Salesforce CRM platform, allowing users to easily access and interpret the data.
In summary, the integration between Einstein Analytics and Salesforce CRM allows businesses to access, analyze, and visualize data from within the Salesforce CRM platform, providing valuable insights into business performance and trends.
What are some common use cases for Einstein Analytics?
Einstein Analytics is a cloud-based business intelligence and analytics platform that is part of the Salesforce platform. It is designed to help organizations make data-driven decisions by providing them with advanced analytics capabilities and data visualization tools. Some common use cases for Einstein Analytics include:
- Sales Performance: Sales organizations can use Einstein Analytics to track their sales performance and gain insights into which products are selling well, which territories are underperforming, and which sales reps are performing best.
- Marketing Effectiveness: Marketers can use Einstein Analytics to track the effectiveness of their marketing campaigns and gain insights into which channels are driving the most conversions and which campaigns are delivering the best ROI.
- Customer Insights: Customer service and support teams can use Einstein Analytics to gain insights into customer behavior and preferences, such as which products are most popular and which customers are most likely to churn.
- Financial Planning and Analysis: Finance teams can use Einstein Analytics to track key financial metrics, such as revenue, expenses, and profit margins, and use the data to make informed decisions about budgeting and forecasting.
- Supply Chain Optimization: Supply chain teams can use Einstein Analytics to track key supply chain metrics, such as inventory levels, order fulfillment times, and shipping costs, and use the data to optimize their supply chain operations.
- Human Resources: HR teams can use Einstein Analytics to track key HR metrics, such as employee turnover, headcount, and diversity metrics, and use the data to make informed decisions about talent management and employee engagement.
These are just a few examples of the many use cases for Einstein Analytics. The platform can be customized and tailored to meet the specific needs of each organization, making it a versatile and powerful tool for making data-driven decisions.
Can you walk us through the steps involved in building a dashboard in Einstein Analytics?
Building a dashboard in Einstein Analytics involves several steps:
- Data Preparation: The first step is to ensure that the data is ready for analysis. This involves preparing the data, cleaning it up, and making sure that it is in the correct format for analysis.
- Data Upload: Once the data is prepared, the next step is to upload it into Einstein Analytics. This can be done using a data upload tool or by connecting to a data source.
- Data Modeling: After the data is uploaded, the next step is to model it. This involves creating relationships between the different data sets and defining the measures and dimensions that will be used in the analysis.
- Data Exploration: Once the data is modeled, the next step is to explore it. This involves creating charts, graphs, and other visualizations to help understand the data and identify any patterns or trends.
- Dashboard Design: The next step is to design the dashboard. This involves choosing the visualizations that will be used, arranging them on the dashboard, and customizing the look and feel of the dashboard.
- Data Refinement: If necessary, the data can be refined during the dashboard design process. This may involve adding additional data sets or modifying the data model.
- Dashboard Deployment: Once the dashboard is designed, the final step is to deploy it. This involves publishing the dashboard and making it available for others to view.
- Dashboard Maintenance: Finally, it is important to regularly maintain the dashboard. This may involve updating the data, modifying the dashboard design, or adding new visualizations.
These are the basic steps involved in building a dashboard in Einstein Analytics. The process can be complex and time-consuming, but with the right tools and expertise, a dashboard can be created that provides valuable insights and helps drive business decisions.
What is Einstein Discovery and how does it relate to Einstein Analytics?
Einstein Discovery is a product offered by Salesforce, a cloud-based customer relationship management (CRM) platform. It is part of the Salesforce Einstein AI platform and is designed to provide businesses with advanced data analysis and predictive insights. Einstein Discovery allows users to automate the process of discovering patterns and relationships in their data, making it easier to understand and make decisions based on that data.
Einstein Analytics, on the other hand, is a platform that enables organizations to analyze their data in real-time, making it possible to quickly gain insights and make data-driven decisions. Einstein Analytics is designed to be user-friendly, making it accessible to businesses of all sizes.
Einstein Discovery and Einstein Analytics are related because Einstein Discovery is a key component of Einstein Analytics. Einstein Discovery provides the predictive insights that are integrated into Einstein Analytics, allowing users to quickly gain insights from their data and make data-driven decisions. This integration makes it possible for organizations to gain a complete understanding of their data and to use that information to make informed decisions, leading to improved business outcomes.
Can you give an example of how Einstein Discovery can be used to solve a business problem?
Suppose a company is trying to identify the factors that drive sales of its products. The company has data on customer demographics, product features, and past sales. By using Einstein Discovery, the company can perform predictive analysis on this data to identify which factors are most important in driving sales.
Einstein Discovery can use machine learning algorithms to analyze the data and identify patterns and relationships between the variables. For example, the analysis might show that a certain age group of customers is more likely to purchase a particular product, or that a specific feature of a product is more appealing to customers.
Using this information, the company can then make informed decisions about how to target its marketing and sales efforts. For example, it might choose to focus its advertising on the specific age group that is most likely to purchase its products, or it might highlight the product features that are most appealing to customers.
In this way, Einstein Discovery can help the company solve a business problem by providing valuable insights into its sales data, which can be used to inform business decisions and drive success.
How does Einstein Discovery handle data preparation and modeling?
Data preparation in Einstein Discovery involves cleaning and transforming the raw data into a format that can be used for modeling and analysis. This includes tasks such as:
- Data integration: Combining multiple data sources into a single data set that can be analyzed.
- Data cleansing: Removing or correcting data errors, inconsistencies, and outliers.
- Data enrichment: Adding additional data to the data set that provides context or additional insights.
- Data transformation: Converting data from one format to another, such as from unstructured to structured data, or from a transactional data format to a summary data format.
Once the data has been prepared, Einstein Discovery can then be used for modeling and analysis. This involves using machine learning algorithms to identify patterns and relationships in the data and build predictive models.
Einstein Discovery uses a combination of supervised and unsupervised machine learning algorithms to perform data modeling and analysis. Some of the algorithms used include:
- Linear regression: Used to predict continuous variables, such as sales or customer lifetime value.
- Logistic regression: Used to predict binary outcomes, such as whether a customer will churn or not.
- Decision trees: Used to model complex relationships between inputs and outputs, such as predicting customer behavior.
- Clustering: Used to group similar data points together and identify patterns and relationships within the data.
Einstein Discovery also provides an intuitive interface for building, testing, and deploying predictive models. This makes it easy for users to build and refine models without needing deep technical knowledge of machine learning algorithms.
In summary, Einstein Discovery handles data preparation and modeling by cleaning and transforming raw data, using machine learning algorithms to identify patterns and relationships, and providing an easy-to-use interface for building and deploying predictive models.
How do you ensure data security and privacy when using Einstein Analytics and Discovery?
Ensuring data security and privacy when using Einstein Analytics and Discovery requires a combination of technical measures, processes, and best practices. Some of the key steps to take include:
- Access controls: It is crucial to implement strong access controls to ensure that only authorized users can access the data. This can be done by setting up authentication and authorization mechanisms, such as Single Sign-On (SSO), and enforcing role-based access.
- Data encryption: Data encryption helps protect sensitive information in transit and at rest. Einstein Analytics and Discovery should be configured to use encryption technologies, such as SSL/TLS and AES encryption, to secure data in transit and storage.
- Data masking: Data masking is a technique used to protect sensitive information by replacing it with fictional data. This technique can be used to protect sensitive data, such as social security numbers, credit card information, and other confidential information.
- Regular backups: Regular backups ensure that data can be recovered in case of a disaster or data loss. Einstein Analytics and Discovery should be configured to perform regular backups to ensure data recovery and minimize the risk of data loss.
- Monitoring and auditing: Regular monitoring and auditing of the data and systems helps detect and prevent security breaches and data theft. Einstein Analytics and Discovery should be configured to perform regular monitoring and auditing, and to alert administrators in case of any suspicious activity.
- Policy enforcement: It is important to enforce policies and procedures to ensure that data security and privacy are maintained. This includes training users on best practices, conducting regular security assessments, and implementing security awareness programs.
- Compliance: It is essential to ensure that the data security and privacy practices are compliant with relevant regulations, such as the General Data Protection Regulation (GDPR), Payment Card Industry Data Security Standard (PCI DSS), and others.
By implementing these measures, organizations can ensure that the data is secure and protected when using Einstein Analytics and Discovery.
What is the role of the Salesforce Einstein Analytics and Discovery Consultant?
A Salesforce Einstein Analytics and Discovery Consultant is responsible for helping organizations harness the power of Salesforce Einstein Analytics and Discovery to achieve their business goals. This person must have a deep understanding of the platform’s features and capabilities and be able to apply that knowledge to help clients solve complex business problems.
Some of the key responsibilities of a Salesforce Einstein Analytics and Discovery Consultant include:
- Analyzing business requirements: The consultant will work with the client to understand their business objectives and goals and determine how Salesforce Einstein Analytics and Discovery can help achieve those objectives.
- Developing dashboards and reports: The consultant will create dashboards and reports to provide clients with real-time insights into their data and help them make informed decisions.
- Data integration: The consultant will help clients integrate their data from multiple sources into Salesforce Einstein Analytics and Discovery, ensuring that all data is properly consolidated, cleaned, and transformed.
- Customizing the platform: The consultant will work with the client to customize the platform to meet their specific needs, including setting up custom metrics, creating custom fields, and creating custom visualizations.
- Training and support: The consultant will provide training and support to clients to help them effectively use Salesforce Einstein Analytics and Discovery, ensuring that they are able to get the most out of the platform.
Overall, the role of a Salesforce Einstein Analytics and Discovery Consultant is critical to helping organizations unlock the full potential of the platform and achieve their business goals. This person must have excellent technical skills, strong communication skills, and a deep understanding of the Salesforce Einstein Analytics and Discovery platform.
What are the key technical skills required for a Salesforce Einstein Analytics and Discovery Consultant?
Here are the key technical skills required for a Salesforce Einstein Analytics and Discovery Consultant:
- Salesforce Platform: A Salesforce Einstein Analytics and Discovery Consultant should have a strong understanding of the Salesforce platform, including custom objects, fields, reporting, and dashboards.
- Einstein Analytics: This includes proficiency in Einstein Discovery, Einstein Predictive Vision Service, Einstein Predictive Vision API, and Einstein Predictive Vision Models.
- Data Management: The consultant should have strong skills in data management, including data warehousing, data modeling, and data architecture. They should also have experience in working with large datasets and be able to analyze and interpret data quickly.
- SQL: Strong knowledge of SQL is essential for a Salesforce Einstein Analytics and Discovery Consultant. They should be able to write complex SQL queries, including joins, subqueries, and aggregate functions.
- Data Visualization: The consultant should have experience in data visualization and be able to create interactive dashboards and charts that are both visually appealing and easy to understand.
- Business Intelligence Tools: Familiarity with other business intelligence tools, such as Tableau, PowerBI, and QlikView, is also a plus for a Salesforce Einstein Analytics and Discovery Consultant.
- Communication Skills: Excellent communication skills are critical for a Salesforce Einstein Analytics and Discovery Consultant. They should be able to explain complex technical concepts to non-technical stakeholders in a clear and concise manner.
- Project Management: The consultant should have experience in project management and be able to manage multiple projects at once, prioritize tasks, and meet deadlines.
- Salesforce Certifications: Salesforce certifications, such as the Salesforce Certified Einstein Analytics and Discovery Consultant certification, are also important for a Salesforce Einstein Analytics and Discovery Consultant. These certifications demonstrate a commitment to staying up-to-date with the latest Salesforce technologies and best practices.
Basic Interview Questions
1. What is The Dataflow in Salesforce Einstein Analytics and Discovery Consultant ?
The dataflow is an essential component of extracting data and creating datasets in JavaScript Object Notation (JSON). It operates by following a set of instructions that identify which objects and fields require data extraction, as well as the specific dataset names to create. Although the dataflow is capable of joining data together, its primary focus is on extracting data.
2. What is Dataset Builder?
The Dataset Builder is a tool that generates the JSON instructions required to create a dataset and then adds those instructions to the dataflow. After that, the dataflow executes the instructions and builds the dataset.
3. What is Cloud Hub?
CloudHub is an integration platform as a service (iPaaS) that enables users to develop complex cross-cloud integration applications in the cloud. It allows you to create APIs on top of existing data sources, integrate on-premises applications with cloud services, and more.
4. What are Persistent queues?
Persistent queues safeguard against message loss and enable you to distribute workloads across a group of workers. On your application, you can use persistent queues to store data in an input queue to disk.
5. What is Clustering?
Clustering provides scalability, workload distribution, and added reliability to your applications on CloudHub. This functionality is powered by CloudHub’s scalable load-balancing service, worker scale-out, and persistent queues features. You can enable clustering features on a per-application basis using the Anypoint Runtime Manager console when either deploying a new application or redeploying an existing application.
6. How can you provide Horizontal Scalability?
To achieve horizontal scalability, CloudHub allows you to choose the number and size of the workers for your application. This feature provides fine-grained control over computing capacity provisioning, giving you the flexibility to scale up your application to handle higher loads at any time.
7. How can you enable and disable either or both features of clustering?
To enable or disable either or both features of clustering, you can either do so when deploying an application to CloudHub for the first time using the Runtime Manager console or by accessing the Deployment tab in the Runtime Manager console for a previously deployed application.
8. How can you build your Dataflow Logic?
You can use Dataflow Editor, a point-and-click interface, to build your dataflow logic from scratch. Here you can add transformations to determine what source data to use, how to transform that data, and which datasets to load the results into.
9. What are transformations and what does Augment Transformation do?
A transformation is the manipulation of data, which can be added to a dataflow to extract data, transform datasets, and more. Augment Transformation, on the other hand, adds columns to a dataset from another related dataset, allowing queries across both related input datasets.
10. What is computeExpression Transformation?
The ComputeExpression Transformation enables you to add derived fields to a dataset based on SAQL expressions, while ComputeRelative Transformation performs calculations based on the previous and next values of the same field in other rows. However, multivalue fields aren’t supported in ComputeExpression Transformation.
11. What is the difference between computeExpression and computeRelative transformations?
The computeExpression and computeRelative transformations are mostly similar, but they have a key difference. The computeExpression transformation performs calculations based on other fields within the same row. The computeRelative transformation performs calculations based on the previous and next values of the same field in other rows.
12. Does computeExpression transformation support multi-value fields?
The Multivalue fields aren’t supported. The dataflow fails if you include a multivalue field in the SAQL Expression parameter.
13. What are Delta Transformations?
Delta Transformations calculate changes in the value of a measure column in a dataset over time and generate an output column to store the delta for each record.
14. What is Tableau CRM?
Tableau CRM is a secure, trusted, and scalable tool that provides intuitive access to data insights through its point-and-click visual interface.
15. When do you implement row-level security on a dataset?
Row-level security on a dataset is implemented when access to records needs to be restricted, by using sharing inheritance and security predicates. Sharing inheritance applies a Salesforce object’s sharing logic to the dataset’s rows, while security predicates are manually assigned filter conditions that define dataset row access.
16. How do you create an Amazon Redshift Connection?
- In Tableau CRM, click the gear icon and select Data Manager.
- In the data manager, click the Connect tab.
- Click Connect to Data.
- Click Add Connection.
- Click the connector’s icon and enter its settings, as described in the Connection Settings section.
- When you finish entering the settings, either click Save or Save & Test. Save & Test validate your settings by attempting to connect to the source. If the connection fails, Tableau CRM shows possible reasons.
17. What should you keep in mind while Migrating Tableau CRM Assets with an Ant Script?
When it comes to migrating Tableau CRM assets using an Ant script, there are some important things to keep in mind. One crucial consideration is that if you are migrating a dashboard with conditional formatting, you will need to manually add the corresponding asset XMD to the project manifest before deploying it to the target org. This is because the asset XMD contains the specific conditional formatting settings for the dashboard, and it is not automatically included in the package.xml file.
18. What are the packages in Tableau CRM Assets?
A package is a container for something as small as an individual component or as large as a set of related apps. Packages can be distributed to other Salesforce users and organizations, including those outside your company. While packages come in two forms—unmanaged and managed—Tableau CRM supports only managed packages, which must be created from a Developer Edition organization.
19. How do you control visibility in an app?
Another important aspect of Tableau CRM is the Asset Visibility feature, which allows App Managers and Editors to control the visibility of select assets. However, it’s important to note that users who only have ‘Viewer’ sharing access will have limited visibility of these assets. App Managers and Editors will still be able to see them regardless of their sharing access.
20. How do you enable Annotations on Dashboard Widgets?
To enable annotations:
- From Setup, enter Feed Tracking in the Quick Find box, and then select Feed Tracking under Chatter.
- Scroll down and select Analytics Asset.
- Select Enable Feed Tracking.
- Click Save.
21. What are Dashboards?
Dashboards are an essential tool for understanding business trends and making data-driven decisions. They allow you to easily identify patterns, track performance and measure the impact of your activities. It’s important to review basic dashboard concepts before building, reading, or sharing them.
22. How does Dashboard sort data?
Dashboard components support two-level sorting. So you can sort by Stage and then by Sum of Amount, or by Average Age and then by Record Count.
23. What are Calendar Heat Map Charts?
If you’re looking to visualize recurring discrete activities over a long period of time, you might want to consider using a Calendar Heat Map Chart. This type of chart allows you to change the granularity of the time-based grouping, from month to week or day, for example.
24. What does Timeseries do ?
One powerful feature of Tableau CRM is Time series, which uses existing data to predict future data points. This tool selects the forecasting model that provides the best fit and can detect seasonality in your data. You can let Timeseries choose the best model or specify the one you want.
25. Can you facet queries with multiple datasets?
When working with multiple datasets, you can use a query in a widget and then facet the query. The query will receive filters from all its datasets and broadcast selections from the first loaded dataset.
26. What are interactions?
Interactions, formerly known as bindings, allow you to specify relationships between different queries on your dashboard. These bindings can modify a query, set an initial filter selection, or change the appearance of a widget dynamically.
27. What is Fuzzy Matching in Salesforce Einstein Analytics and Discovery Consultant?
Fuzzy Matching is a feature of Salesforce Einstein Analytics and Discovery Consultant that adds uniformity to spelling variations in variables. This helps to improve categorizations and predictions by ensuring consistency in the data.
28. What happens when you impute missing numerical values in your dataset?
Einstein Discovery allows you to impute missing numerical values in your dataset. Enable statistical imputation on a numeric variable, and Einstein Discovery automatically replaces missing values with data derived from another subset of your data.
Get ready to prepare for Salesforce Einstein Analytics and Discovery Consultant now!
Take the Salesforce Einstein Analytics and Discovery Consultant Free Practice Tests Now!